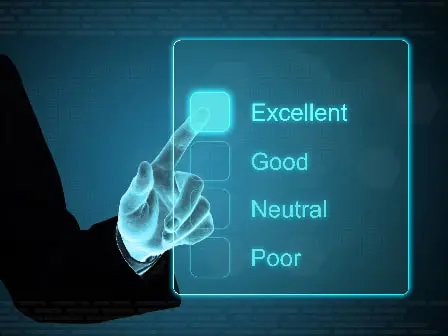
We recently published an ebook titled “Data Governance: An Executive’s Survival Guide”. The following is a sampling of the chapter on data quality.
The Value of Data Quality
Data is the lifeblood of modern organizations, providing crucial insights that can drive decision-making and innovation. However, the value of data is only as good as its quality. Poor quality data can lead to costly mistakes, misinformed decisions, and reputational damage. That's why it's essential to ensure your organization's data fits its intended purpose.
Data quality is a critical aspect of data governance. It refers to the accuracy, completeness, consistency, and relevance of data. In other words, data quality measures how well data meets its intended purpose. Good quality data is reliable, up-to-date, and trustworthy and can drive meaningful insights and actions.

The 5 Characteristics of Good Data Quality
There are 5 key characteristics of good data quality that organizations should consider when managing their data.
Accuracy: Good quality data should accurately reflect the event or object that it describes. If the data is inaccurate, it can lead to wrong conclusions and costly mistakes. It's essential to ensure that the data is checked for accuracy regularly.
Completeness: Good quality data should fulfill certain expectations of comprehensiveness within the organization. Ensuring the data is complete enough to draw meaningful conclusions is vital. Incomplete data can also lead to vague insights and decisions.
Consistency: Good quality data should be consistent across multiple and separate data sets. If there are inconsistencies in the data, it can lead to confusion and errors. Consistency doesn't require the data be correct, but it’s still necessary for good data quality.
Integrity: Good quality data should comply with the organization's data procedures and validation. Data integrity ensures that the data has no unintended errors and corresponds to appropriate data types. It's essential to establish a data validation process to ensure the integrity of the data.
Timeliness: Good quality data should be available when users need it. If the data isn’t available on time, it can lead to missed opportunities and poor decision-making. Organizations should ensure their data is up-to-date and readily available when needed.
By ensuring your data meets these 5 characteristics of good data quality, you can ensure your decisions and insights are based on accurate, complete, consistent, and trustworthy data.
Metrics for Measuring Data Quality Efforts
Measuring data quality is essential for organizations that rely on data for decision-making. There are 5 metrics organizations can use to evaluate their data quality efforts.
Ratio of data to errors: Track the number of errors found within a data set corresponding to the actual size of the set. The goal would be to minimize the number of errors and ensure the data is accurate and trustworthy.
Number of empty values: Count the number of times an empty field exists within a data set. Empty values indicate missing information or information recorded in the wrong field, which can lead to incorrect insights and decisions.
Data time-to-value: How long does it take to gain meaningful insights from a data set? The shorter the time-to-value, the more valuable the data is to the organization.
Data transformation error rate: How often does a data transformation operation fail? Data transformation errors can lead to incomplete or incorrect data, negatively impacting decision-making.
Data storage costs: Storing data without using it can often indicate that it’s of low quality. However, if the data storage costs decline while the data stays the same or continues to grow, the data quality is likely improving.
By measuring these 5 metrics, organizations can evaluate the effectiveness of their data quality efforts and identify areas for improvement. Ultimately, the goal is to ensure the data is accurate, complete, consistent, and trustworthy, and can be used to drive meaningful insights and decisions.
The journey toward adequate data quality and management requires ongoing effort and commitment, but the benefits of good data quality are well worth the investment. With the right tools, strategies, and mindset, organizations can unlock the full potential of their data and drive success in today's data-driven world.